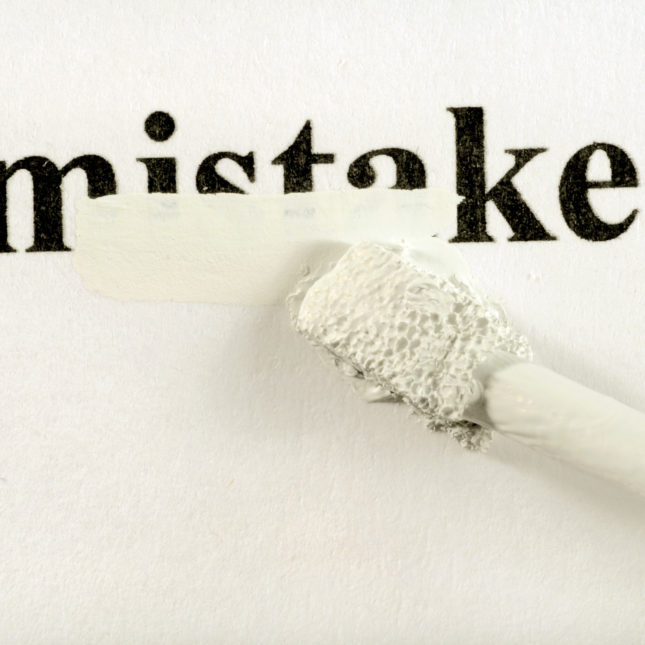
Five sentences. Longer than a tweet but shorter than the average elementary school essay. That’s the length of an influential letter published in 1980 in the New England Journal of Medicine, the world’s most prestigious medical journal. It alleged that narcotics are not addictive. This letter, combined with aggressive marketing efforts by pharmaceutical companies and the emergence of improving pain control as a focus for physicians and hospitals, led doctors to begin prescribing opioids as painkillers for conditions that once simply called for aspirin.
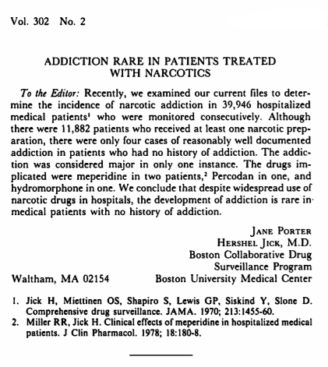
The outcome of this perfect storm? Millions of Americans addicted to opioids, and more than 200,000 dead by overdose between 1999 and 2016. And that number keeps growing.
Why was such a short letter so influential? It has what are considered to be the hallmarks of a reliable scientific publication: It appears in a prestigious journal and it has been highly cited by other researchers, 608 times as of 2017.
When a group of researchers analyzed the hundreds of papers citing the letter, their findings prompted NEJM to append the letter with a stark warning: “For reasons of public health, readers should be aware that this letter has been ‘heavily and uncritically cited’ as evidence that addiction is rare with opioid therapy.”
This is just one example that science, like news and social media, has a “fake news” problem.
Major pharmaceutical organizations have published reports and given talks saying that they are unable to validate studies, sometimes up to 90 percent of the times. The Open Science Collaboration turned up similar worrying results, showing that the majority of psychology studies can’t be confirmed. One initiative that tried to replicate the top 50 cancer studies had to stop at 18, simply because it was too difficult and expensive to continue. Irreproducibility in the sciences seems to be one of the most reproducible findings, perhaps because each irreproducible study generates many more that follow it.
The issue of results becoming “facts” by being repeated citation by citation isn’t a new plague for science — or the internet. In fact, it has been a focus since the beginning of search engines. In the early days of ranking webpages, the more that other sites linked to yours, the more your ranking was boosted. In science today, the more a paper is cited, the more visible it is and the better it is perceived.
Google revolutionized webpage ranking with PageRank, an algorithm that not only evaluated the number of inbound links, but also the quality of those links (it evaluated quality by determining the number of links those links themselves had received).
A somewhat similar ranking, known as Shepardizing, has been available to the legal community through the LexisNexis database. It lets lawyers easily understand and cite cases that still stand, as well as those that have been overturned or contested.
Science currently has no good way to assess scientific rigor, and proxies of quality — such as social media attention, total citation counts, and journal brand — are highly flawed, if only because a citation refuting a report is counted the same way as one supporting it.
That may be changing. With today’s progress in machine learning and artificial intelligence, machines can do laborious tasks like citation analysis with great accuracy and efficiency. Unlike the internet, though, most research cannot be easily scraped or text mined because it is behind paywalls and bound by restrictive copyrights. This means it is easier to text mine Twitter than it is to text mine the cancer literature.
Opening up scientific publications to read and reuse, referred to as open access, has been a growing movement over the last decade. It now seems to be at a tipping point, with funders in the European Union proposing to mandate by 2020 that all future publications be open access immediately upon publication. The consequences of that change could be profound for science and society.
Deep learning models, used to power advances in self-driving cars and voice recognition (hi, Siri!), tend to emerge from communities that openly share their papers, data, and code. If this can be expanded to the biomedical research literature, the potential for new advances is far-reaching.
The company I co-founded, scite, is working to develop a system that uses natural language processing combined with a network of human experts to classify citations as supporting, contradicting, or simply mentioning. Such a system has the potential to stop publications like the now-infamous 1980 letter on opioids from becoming authoritative by shifting the emphasis to how research has been cited, not just how many times it has been cited. Science has had intense interest in reproducibility initiatives and proposed policy changes, but to the best of my knowledge no one is doing what scite is trying to do.
Marc Andreessen, the co-author of Mosaic and the co-founder of Netscape, once wrote that “software is eating the world.” It’s taken a bit longer than expected, but it’s now gobbling up scientific literature.
Josh Nicholson is the CEO and co-founder of scite.
To submit a correction request, please visit our Contact Us page.
STAT encourages you to share your voice. We welcome your commentary, criticism, and expertise on our subscriber-only platform, STAT+ Connect